Credit Scoring Using Alternate Data
August 21, 2018
In
ANALYTICS
Access to centralized, accurate credit histories is taken for granted in many parts of the world, however, that is not the case in India, wherein nearly 80% of the adult population does not have a formal credit record. As a result of this, a majority of the population faces difficulty in accessing formal credit, even though many of them are creditworthy. The fact that they work in the unorganized sector, have temporary jobs or do not have so-called steady sources of income, serves to be an impediment, in this case.
Apart from this, lending to lower-income households or small/informal enterprises also is a challenge, as many of them are barely familiar with formal financial services, which, in turn, limits their ability to make sound decisions regarding, responsible/appropriate use of credit. Hence, lenders in such scenarios have insufficient data that might traditionally help them with lending decisions (e.g. official proof of income, credit history etc.)
In the aforementioned scenarios, there are two options or business models that the lenders employ:
• Traditional consumer finance, wherein higher interest rates compensate for higher default incidence, supplementing revenue with penalties, other fees etc.
• Microcredit, which focuses on informal microenterprises, with short-term loans, labor intensive relationship between borrowers and loan officers, using joint liability groups, continuous lending cycles etc.
Neither of these models is ideal or cost-effective when talking about the plethora of needs of economically active lower-income families, youth and businesses, in terms of sustainability. This is where the opportunity for alternate data comes in, where data analytics can be leveraged to create better risk models.
The rapid proliferation of phones/internet/social media and the explosion of e-commerce are proving to be a game changer. India has about 462 million Internet users out of which 153 million are active on social media/e-commerce platforms, resulting in increased smartphone penetration that has created a digitally visible segment.
Data analytics hence, looks at the following progressions:
1. Identification of promising data sources
2. Securing access to the appropriate data
3. Converting data into credit insights
These involve looking at data sources, ranging from transactional (phone usage pattern, utility-bill payment history etc.), locational, social profiles (social scraping), to behavioral markers (psychometric tests etc. in the case of rural income bases), to assess the risk of an individual. Running of basic searches around the internet is further made, for the verification of an applicant’s employment, spending habits, cash withdrawal rates etc. basically to establish a consistency of behavior that would demonstrate stability, applicable for first-time borrowers.
Advantages of Using Alternate Credit Scoring:
There lie several growth opportunities for market players, looking at alternative credit scoring, namely:
• A New Segment of Customers: The current section of the population that is unable to attain a formal valid credit score includes, young professionals, homeowners, retirees and new immigrants.
• A Differentiated Customer Experience: Apart from the expansion of tailored products and relatively outgoing customer experience, alternate credit scoring can help the lender in streamlining and automating the loan reimbursement process, delivering faster loans to customers in the process.
• Enhancement of Underwriting Processes: The traditional practice of underwriting can be improved via non-traditional/non-conventional variables, such as credit-card/banking transactions, social scraping, employment history etc. This will, therefore, lead to a reduction in credit risk via improved risk modelling/monitoring.
Recent times have seen numerous Fintechs coming to fore, seeking to capitalize on this opportunity, using proprietary ‘machine-learning’ algorithms in sifting through the multitude of data points that are available to consumersIL&FS Technologies too has developed models for credit scoring using alternate data, which can eventually develop into a perfect solution for financial inclusion.
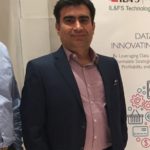