Data Analytics and Fraud Prevention: An Insight
August 21, 2018
In
ANALYTICS
As the world economy and our lives rapidly move on to digital platforms, government and financial organizations worldwide are facing new sets of problems, in trying to protect the interest of citizens/customers from known and unknown threats. Fraud, by definition, encompasses a wide range of illicit practices and acts of misrepresentation or intentional deception. Perpetrated by organizations/individuals to obtain money, property/services or to avoid payment/loss of services; or to even ensure personal/business advantages; the financial and operational impact of frauds can be staggering.
Technology enablement has made data available in a more structured form for analytical purposes, however, organizations today (both public and private sector), are not capitalizing on the development enough and are not performing proactive monitoring of data for fraud indicators, limiting the analysis to the quantification of the impact on a financial basis, when fraud is detected via some other means. Thus, this brings us to the topic at hand:
The Need for Data Analytics in Fraud Prevention:
• Improved Efficiency: The detection and monitoring of potentially fraudulent behaviour, becomes automated.
• Repeatable Tests: Fraud tests via analytics are repeatable and can be run on the data at any given hour.
• Wider Scope of Coverage: Instead of spot checks on a transaction, full coverage is granted of the testing population, hence the chances of finding exceptional items is much higher.
• Early Warning System: Quick identification of potentially fraudulent behaviour, can help in taking preventative measures before the fraud becomes material.
Analytical techniques such as the calculation of statistical parameters, classification and stratification of numbers, duplicate/gap testing, the summation of numeric values, validation of entry dates etc. are quite effective when it comes to fraud detection, both incidental and accidental and can help control revenue leakage/decay.
Taking an example of the public sector, governments are charged with the responsibility of providing services that are for the public good, in limited fiscal means. Having access to public funds makes the government a target to fraudulent activities, from both within as well as the public, making fraud a high-risk area, featuring more prominently in government audit plans as opposed to those in private sector. Be it corruption in the form of bid-rigging/invoice kickbacks, benefits fraud, customs evasion, tax fraud etc. or other billing/expense reimbursements, grants mismanagement etc., the ultimate sufferer as a result of these malpractices happens to be the honest, tax-paying citizen. Hence the need for the application of data analytics for detection and prevention, is quite pressing in the public sector.
ITL has in fact, proactively worked with the governments of Rajasthan and Tamil Nadu, implementing fraud monitoring programs across various departments, putting together a central copy from reduplicated data, ensuring that the working government can provide maximum citizen benefit, with public funds from various schemes, reaching the intended recipient.
While the benefits of data analytics are plenty when it comes to measures of fraud prevention, there are challenges associated with it that must be recognized:
Challenges Faced by Data Analytics in Fraud Prevention:
• Data Quality: The results that are received from the analytics tests are dependent, on the quality of data input. Hence, it is quite important to assess the quality of data and if necessary, perform validation/cleansing.
• Data Volumes: There are instances when the data volume for certain business processes, may be significant in quality. It is thus important that the data analytics infrastructure, be capable of handling such volumes.
• Data Security: Appropriate security controls need to be considered throughout the extraction and analysis, in order to protect the integrity and confidentiality, of the source data.
Hence, overcoming these challenges, instead of relying on reactive measures, organizations should opt for a more hands-on approach, when it comes to fraud detection/prevention.Instead of retrospective analysis, constant monitoring of transaction can help an organization detect potentially fraudulent transactions on a daily/weekly/monthly basis and eventually narrow down on bands of transactions/areas, which pose particularly high risks.
Thus by leveraging the power of data analytics, organizations can mitigate the negative impact that fraud incurs, due to significant losses in revenue for financial/corporate institutions and the public sector alike.
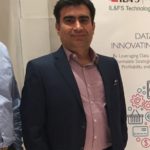