Predictive Customer Churn Analysis Modelling in Banking Sector
August 27, 2018
In
ANALYTICS
In today’s competitive commercial arena, customer-retention has become a pressing issue for banks, with heightened competition and increased regulations making it difficult to stand apart from the sea of available options. Churn is a major problem in the banking sector today, as the process of losing customers and then having to acquire new customers can turn out to be very expensive. Studies have in fact shown, that banks can increase their profits by almost up to 85%, just by improving their retention rates by up to 5%.
The amount of raw data that is stored in banking databases is massive in volume and constantly increasing. Using technologies like data mining, it is possible to uncover patterns and relationships between data, so as to ameliorate business decision processes. Thus, bringing together of various techniques like data mining, pattern recognition, machine learning, statistics, knowledge acquisition, neural networks etc. can be used for business insights like fraud detection, market analysis, credit analysis and the topic at hand – customer churn analysis.
What is Customer Churn Analysis?
Customer churn as a banking term, denotes the movement of customers from one bank to another, closing all of his/her accounts and stopping business with the previous bank. Though the reasons may range anywhere from convenience to better facilities, the real problem, however, lies in the fact that banks do not always have access to feedback data, which could help in the assessment and prevention of churning behaviour.
Thus, in order to make accurate assessments/predictions on the basis of historical data and finding patterns/linkages between likely churners and existing customers, the need for creating predictive churn analysis modelling via leveraging data analytics
Predictive Data Mining:
Described as a process of exploring large data sets, with the objective of discovering meaningful patterns, data mining can be leveraged to uncover data patterns for business advantage. Using predictive classification techniques such as naive Bayes technique, linear discriminant techniques etc., data is classified for churn modelling.
Data mining is an iterative process, with generally four phases:
o The first phase involves problem definition, which means the translation of the specific business problem, into a data mining problem.
o The second phase is the gathering and preparation of data phase, wherein data is transformed into a pre-specified format. Data is then cleansed, which involves the detection/correction/removal of corrupt/inaccurate data records. Data quality is a major concern when it comes to data mining.
o The third phase is the Model building and evaluation phase, in which various modelling techniques are applied, in order to, calibrate parameters to optimal values
o Knowledge deployment is the fourth phase, in which data mining is used in a target environment to organize/present results to the user.
In order to maintain competitiveness in the market, the ability to predict possible churners is important for banks so as to take proactive measures regarding the retaining of loyal customers. Creating an effective/accurate churn prediction model is now an important research problem and additional characteristics like credit return, unauthorized overdraft, savings, monthly consumption etc., can help in creating different models for varying problems. Profiling can help a company take affirmative action to keep customers from leaving, as retaining customers is far less expensive than the acquisition of new ones. Predictive Churn analysis modelling can thus facilitate, a long-term customer strategy for banks to minimize attrition.
IL&FS Technologies has implemented a CASA Customer Churn Prediction model for a Public Sector Bank, which provides a churn prediction score / probability of the bank’s HNI customers. Thus providing the bank with a manageable size list of customers that its customer service team can target to reduce churn. The model also provides much needed insights on the factors that have been contributing to churn of its customers.
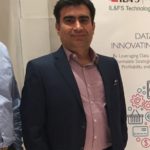