Social Sentiment Analysis for Good Governance
August 27, 2018
In
ANALYTICS
We live in an age of near-ubiquitous communication platforms and advanced analytic capabilities; sentiment analysis of social media, thus, holds huge potential for both the private and public sector. The role of social media, when it comes to promoting good governance, is something that is being rapidly realized by governments/policy makers all over the world. With the vast volume of opinion now available in the form of blogs, discussion forums, news, surveys, review sites, tweets etc. making appropriate belief of thousands of views and the discovery of problems upon their immediate occurrence, is something that opinion mining/sentiment analysis can facilitate.
For governments, social media provides the opportunity to inform/educate citizens, innovate new ways to exceed citizen-expectations and also enable a more effective public workforce in the process. Thus, social sentiment analysis facilitates better possibilities for higher engagement and good governance. The Indian government too has recognized its potential and is trying to profusely use social sentiment analysis towards real-time citizen management.
How Social Sentiment Analysis Works:
• Information Extraction: Most helpful when dealing with sheer volumes of information, information extraction algorithms can identify relationships between acknowledged sequences, to provide significant information.
• Categorization of Opinions: All the information gathered via Information Retrieval (IR) and Information Extraction (IE) tools, is placed into pre-defined topics on the basis of which an attempt to process recognized opinions is made. The categorization of data is generally dependent on the glossary of predefined-topics and the identification of relationships, which is done by looking for wider terms, synonyms, narrower terms etc.
• Clustering: As opposed to using pre-defined topics as in the case of opinion categorization; clustering is used to group data that is similar in some manner. Clustering algorithms create topics for each pool of data and then measure how well various pools of data fit with each other.
• Topic Tracking: Topic tracking generally involves, keeping an eye on user profiles on the basis of the topics that the user generally views and predicting other topics of interest to the user. By utilizing appropriate mining tools, topic tracking systems can be optimized to automatically infer a user’s interest, on the basis of their reading history or click-through information.
• Summarization: Summarization of data is crucial to determining whether the information meets the user’s requirement. Its basic use lies in shortening the length while retaining the main points, as well as cohesiveness.
Real-time Applicability:
Analytics technology in use today, can facilitate sophisticated and automated predictive analysis and sentiment monitoring. Establishing a taxonomy of crucial keywords, analytic tools and combining it with structured data can help public service departments in the better formulation of outgoing messages, targeting of specific audience for the messages and also efficient addressing of citizen’s complaints.
An optional addition of geo-location layer can also help in mapping issues to their respective locations. In case of crisis, municipal, state-level or even central-level bodied can deploy additional resources if necessary by leveraging clever-data.
The application of predictive analytics and social sentiment analysis are overarching and impactful. Social media, has now become a strategic need, with mere participation in conversation not being enough. The sharing of information, as well as monitoring of social feeds, can make citizen convenience and service delivery much moreefficient.
IL&FS Technologies has developed a framework for Social Sentiment Analysis, using open source technologies. Itis currently being used by a State government to gauge the sentiments of its citizens on – new citizen benefit schemes that are launched, understand and address grievances and thus provide pro-active governance
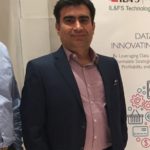